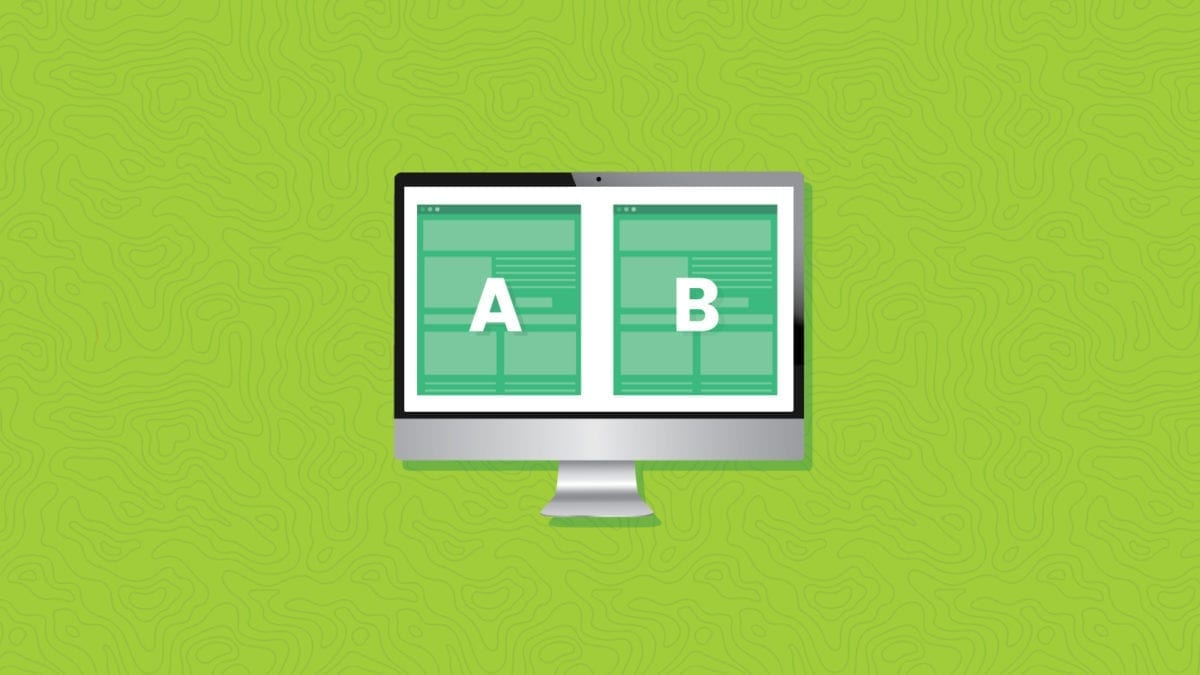
7 Rules to Follow for Email A/B Testing Done Right
The smallest changes in email design, copywriting, or incentives can make a major difference in the performance of a campaign. A different button color, subject line, or send time could determine whether your email is a success or a flop.
Plus, what works for one list of contacts may not resonate with another. Certain segments of subscribers could respond quite differently than others.
This is why it’s critical to test, test, and test your emails some more by split-testing your content, incentives, and send time. In order to run A/B tests that yield repeatable, statistically significant test results, you’ll need to adopt a systematic approach. When done correctly, your A/B tests yield results that’ll actually improve your digital marketing strategy in the long run.
We know this sounds like a lot, so we’ve put together some guidelines to help you get started. In this article, we’ll go over what A/B tests are, what elements you can test, and leave you with seven rules to follow once you start testing.
Table of content
-
01
What is an A/B test? -
02
What email elements can I A/B test? - 1. Subject lines
- 2. Preview text
- 3. Sender name
- 4. CTAs
- 5. Images
- 6. Personalization
-
03
What are seven rules to follow for effective A/B tests? - 1. Know your baseline results
- 2. Test only one element at a time
- 3. Test your elements within the same time frame
- 4. Measure the data that matters
- 5. Test with a large enough list
- 6. Ensure your results are statistically significant
- 7. Don’t stop testing
-
04
Wrapping up
What is an A/B test?
A/B testing (or “split testing”) is a simple way to test:
(Version A) The current design of your web page, email marketing campaign, or ad
against
(Version B) Changes to your current design.
This is done to see which design will produce the most positive results. You’ll use specific email marketing metrics to define a winner. That will depend on the goal of your email. Which version, A or B, drove more opens, clicks, conversions, downloads, or sales?
A/B testing is a crucial part of the email marketing campaign process. A simple tweak in your email campaign or landing page could significantly increase your effectiveness.
Here's an email A/B test example from our friends at Sinch Mailgun. They wanted to know if their subscribers would respond better to a plain text email promoting a white paper or an HTML email with more design elements.
Version A
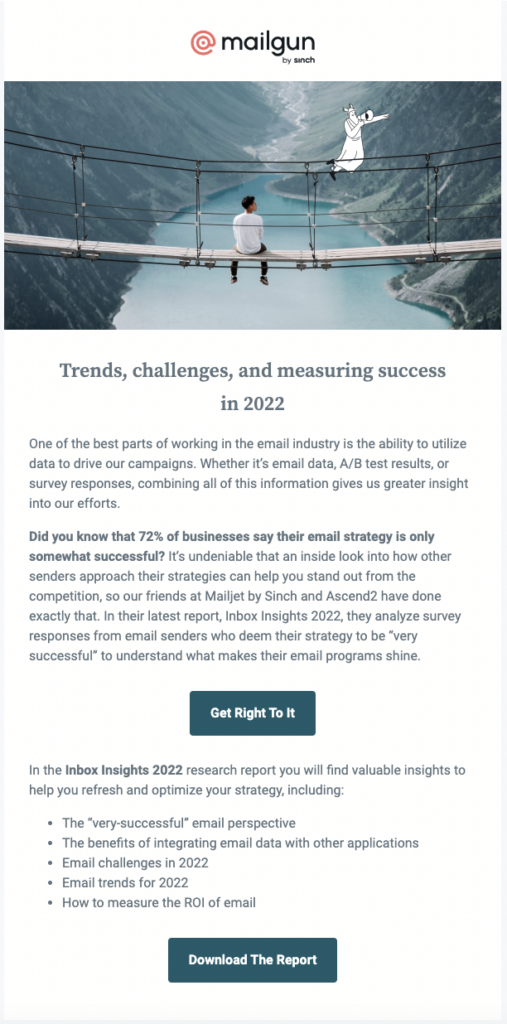
Version B
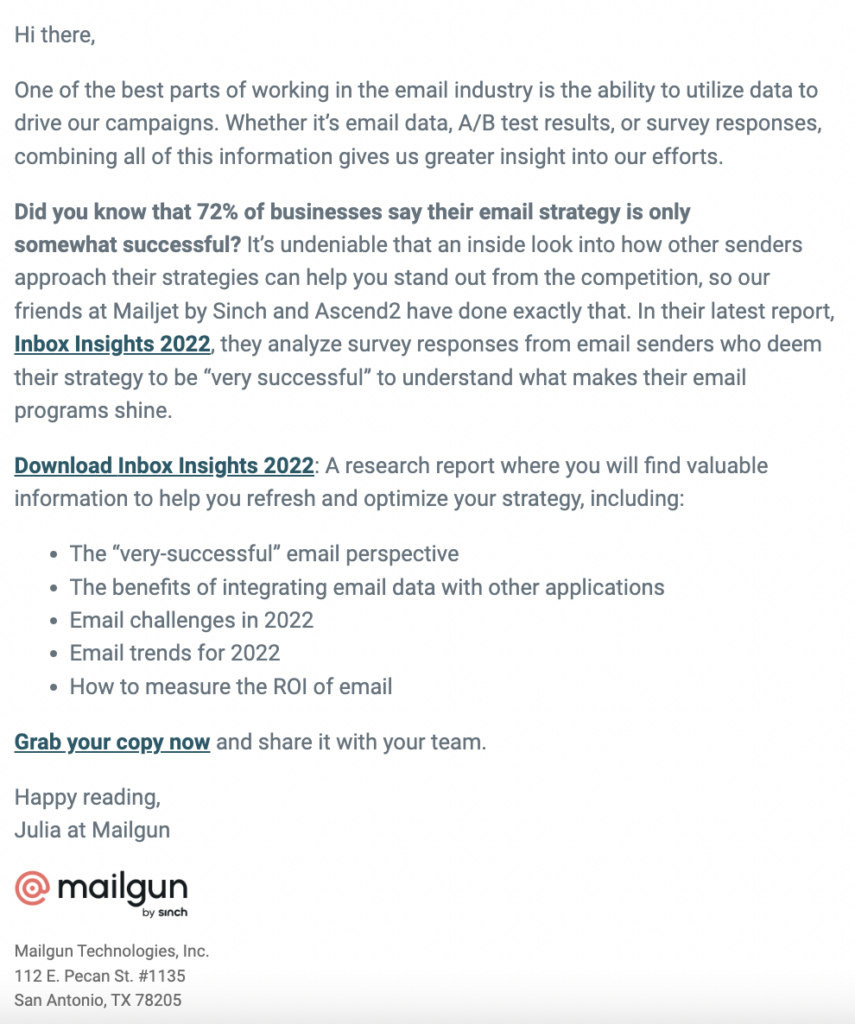
Results were inconclusive on this test, meaning there wasn't a large enough statistical difference between Version A and Version B. That happens now and then with A/B testing. Don't worry about it. It either means that what you're testing doesn't really matter as much as you thought, or it could mean the changes you tested weren't noticeable enough.
What email elements can I A/B test?
Ready to get started? Let’s go over six email elements in your HTML email template that you can A/B test:
- Subject lines
- Preview text
- Sender (“From”) name
- Calls-to-Action (CTAs)
- Images
- Personalization
Let’s dive into each of these below.
1. Subject lines
The inbox is a crowded place, and your subject line is your first chance to grab a subsriber’s attention. You might think your subject line is clever and compelling. But what do your customers and subscribers think? Use A/B testing to try out different subject lines and see if you can find the best fit for your mailing list.
For example, this particular sender decided to A/B test two different ideas, including one that uses an emoji in the subject line.
Version A

Version B

Note: If you get an email from this sender, we highly suggest you mark it as spam.
2. Preview text
If your subject line is the first thing your users see, the preview text is a close second. Check out the following example below:

Ibex uses the preview text space following the subject line to clarify how you can be warm weather-ready. Their new Spring gear is here!
As with subject lines, the preview text can make or break whether your subscriber opens your email. Get a higher open rate by A/B testing different preview text copy and subject line combinations.
3. Sender name
You might not pay too much attention to your sender name (the name in the “From” section), but this might be a place where you want to test out a few different options. For instance, do you want to keep it professional and just have your brand name? Or do you want to make it personal and let your readers know your email is coming from a real person? Check out the two examples from Grist, an independent newspaper, below:


Grist uses different sender names depending on the type of email they’re sending. They opt to use a personal sender name, “Tristan at Grist,” when speaking directly to “you,” the Grist reader. They choose to send from “The Grist Team” when talking about less personal topics, like World Press Freedom Day.
Not sure which works best for your email marketing campaign? A/B test different sender names to see what will bag you the opens you want.
4. CTAs
Once your customer has opened your email, you want to make sure your CTA is attention-grabbing and spurs your subscriber to action. Use A/B testing to see what CTA text will resonate the most with your user base and secure that click-through!
You can test the words used in the CTA, the style and color of CTA buttons, as well as the placement of the CTA within the body of the email. But you should really only test one of these factors at a time. Otherwise, it won't be clear if the color, text, or styles was what convinced people to click. For example...
First A/B test the button copy:
Version A
Version B
When you have a winner, then you can A/B test the button color:
Version A
Version B
Check out our tips for crafting a killer CTA.
5. Images
The right images can supercharge your email marketing campaign. But how do you know you’re using the right images? Does your audience prefer memes or images of a more professional nature? Do your users prefer GIFs or still images? A/B testing should sort you out!
For example, your subscribers may respond better to product images than stock photography. Or, they may engage more when animations in your emails are subtle because too much movement is distracting.
Version A
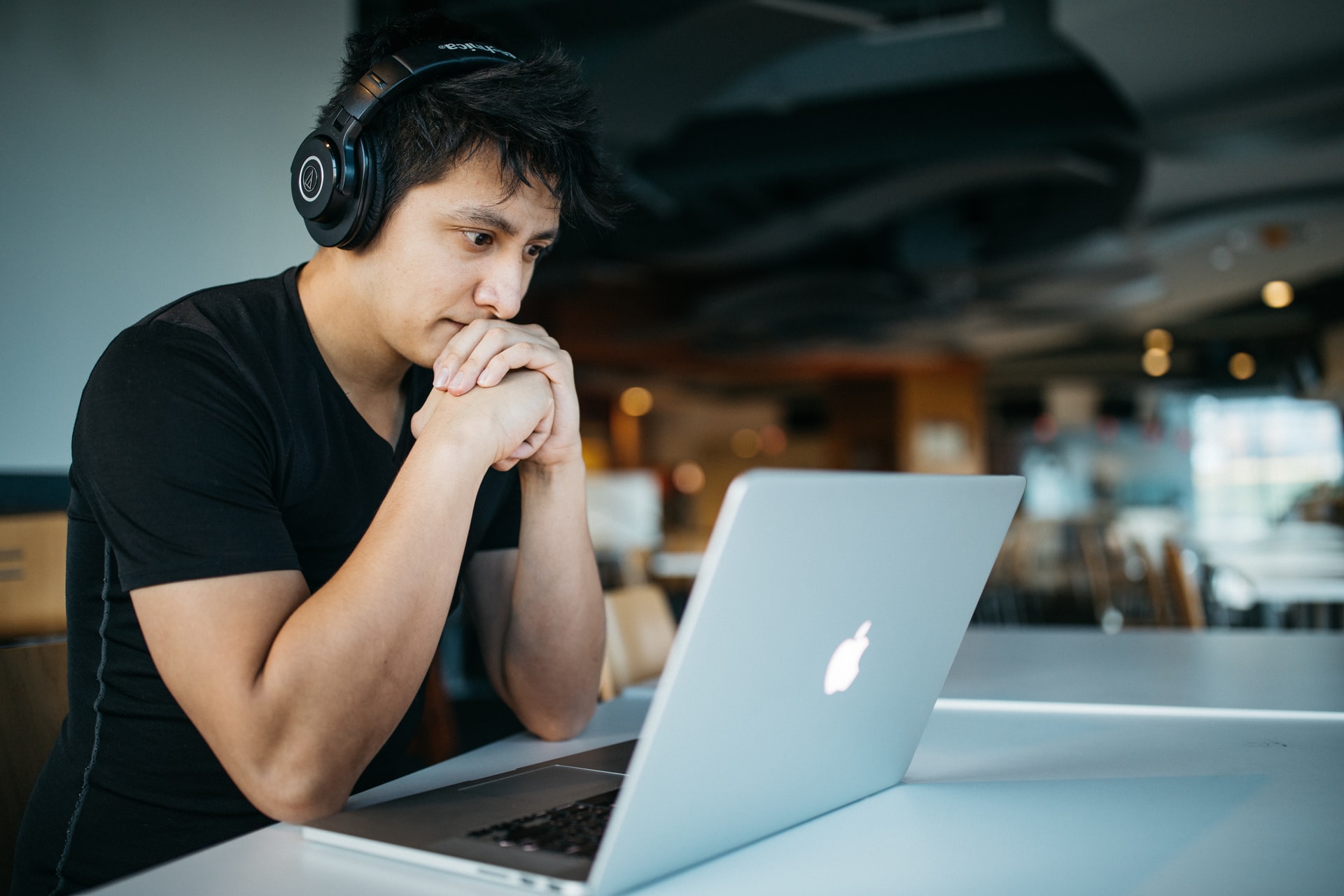
Versions B
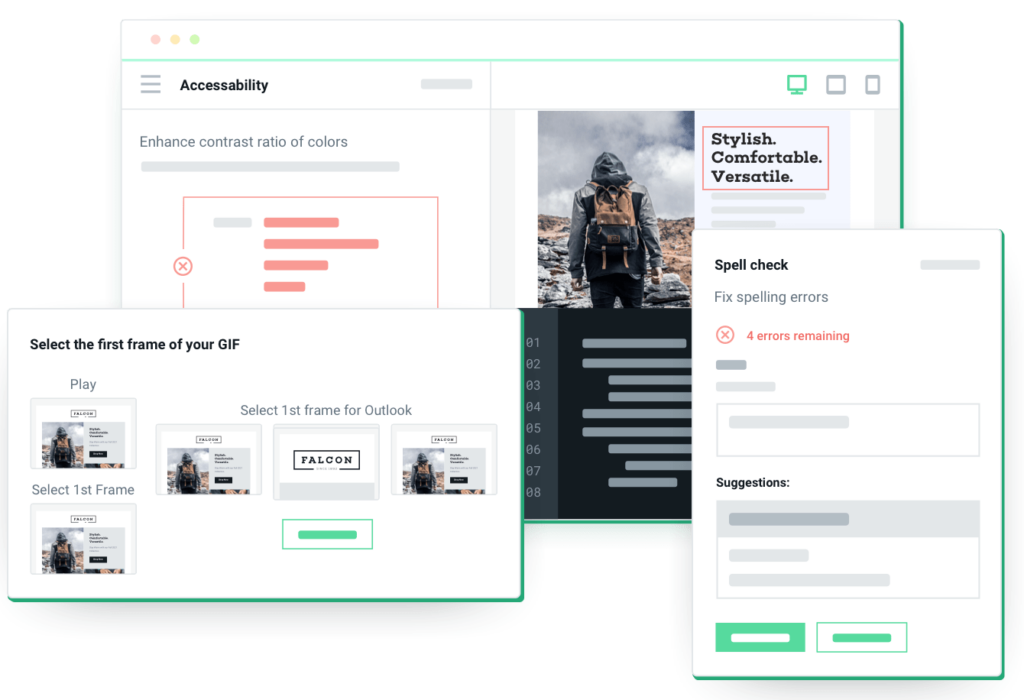
6. Personalization
Using your subscriber’s name in your email is a great start towards personalization, but you can take it a step further with dynamic content. Dynamic content uses automation to create personalization at scale. If you’re not sure where to start, use A/B testing to narrow down the different elements you want to personalize with dynamic content to best serve your customer base.
What are seven rules to follow for effective A/B tests?
Now that we’ve gotten the basics out of the way, here are seven best practices for A/B testing:
- Know your baseline results.
- Test only one element at a time.
- Test all your elements within the same time frame.
- Measure the data that matters.
- Test your whole list, not just a subset.
- Ensure your results are statistically significant.
- Don’t stop testing.
Let’s dig into each of these guidelines below.
1. Know your baseline results
Get a good grasp of your baseline metric to know which of the two versions in your A/B test yielded better-than-normal results. A baseline result means you already know your average open, click-through, and conversion rates.
If you ran an A/B test and your conversion rate for A was 1.3%, and your conversion rate for B was 3.23%, but your baseline conversion rate was 4.5%, the test had a winner, yes, but what you tested won’t positively impact your bottom line in the long run. You want to test versions A and B against each other, but you also want to know that whichever one does better in the test is also doing better than your current results.
2. Test only one element at a time
This is the foundation for yielding successful (and true) results. Don’t test two different layouts and two different CTAs at once. This could mess up your control condition because you’ll have nothing to compare your changes to. If you test multiple elements against each other in an A/B test, you won’t be able to identify the elements that resulted in more opens, clicks, and conversions.
If you do test more than one thing at once, you’re venturing into multivariate testing, which is more complex than email A/B testing because you’ll have more than just two versions.
3. Test your elements within the same time frame
When you are A/B testing an email, two variations of the same test must be run simultaneously. The date and time during which each test is run can drastically impact and skew your results.
If you test one element on Tuesday and another element on Friday, you cannot determine if your results are actually statistically significant. Essentially, the send time brings another variable into the testing equation. How can you be certain subscribers behave the same way on different days of the week or the time of day?
This will be less of an issue when testing the performance of automated email campaigns or transactional emails that are triggered by a specific event or subscriber behavior. In that case, you’ll want to examine the results over an extended time period.
4. Measure the data that matters
As we mentioned above, you can test many different things, like email subject lines, images, email layouts, and CTAs. However, you should remember that each of these elements affects a different part of the conversion process.
For example, if you’re deciding that you’re going to test two different CTA buttons, it wouldn’t make any sense to see which version had the better open rate. Instead, if you’re testing two buttons, the data you will want to hone in on is click-through rate (CTR) or click-to-open rate (CTOR). You would, however, look at which email got better open rates if you were testing two different subject lines.
5. Test with a large enough list
The larger your test sample, the more accurate, repeatable, and reliable your results will be! If you’re only using a small subset of your list, the results may not produce statistically significant results.
There may be times when you want to A/B test how a specific segment of subscribers responds to variables in an email campaign. If the email is meant for a certain target audience, it wouldn’t make sense to split test it with your entire list. However, what you should avoid is split testing the same changes between two different segments of subscribers. If you identify two groups with different characteristics/demographics, you can’t guarantee that your results aren’t skewed based on the make-up of these groups. Handpicking groups can negatively impact your results. You want to gather empirical data – not biased data – to determine which version of your A/B test led to better conversions.
6. Ensure your results are statistically significant
If your results aren’t statistically significant, you’ve failed to design an actionable A/B test. It’s shockingly easy to get results that are due to random chance. ”Statistical significance” coincides with another A/B testing term called “confidence level.” The confidence level is the probability that the measured conversion rate differs from the control page conversion rate for reasons other than chance alone.
You should have a confidence level of at least 90-95% before you can determine that your results are statistically significant. If you had a very low response to an email campaign sent out the day before Christmas, you should consider that the holiday might have negatively impacted your open rates. Numbers are important, but you must also be able to analyze the numbers logically to gain a conclusive summary of the results. Quality trumps quantity any day of the week in A/B testing. If in doubt, run the test again to validate the results!
If you need some help crunching the numbers, grab this A/B calculator to be confident your changes have really improved your conversions.
7. Don’t stop testing
Alright, you’ve done your first set of A/B tests. You’re done now, right?
Nope. Just as your email list is constantly growing and user behavior is constantly evolving, you should keep testing to stay in sync with your audience.
If your marketing KPIs have seen a boost from your A/B tests, figure out what you’re doing right and keep doing that. But don’t just do that – do more. For instance, if switching up your sender name saw a boost in opens, how about optimizing click-throughs with an A/B tested CTA?
Wrapping up
At Email on Acid, testing is at the core of our mission. Before you set up the perfect A/B test for your campaign, there’s a different type of email testing you should never skip. Check out our Campaign Pre-check tool to help you preview emails to be sure they will look fantastic in every inbox. Because every client renders your HTML differently, testing your email across the most popular clients and devices is critical.
Try us free for seven days and get unlimited access to email, image, and spam testing to ensure you get delivered and look good doing it!
This article was updated on August 17, 2022. It was first published in March of 2017.